Player Rating & Matchmaking via Bayesian Inference
Player rating systems are essential nearly to any game situated in different contexts (video games, sports etc).
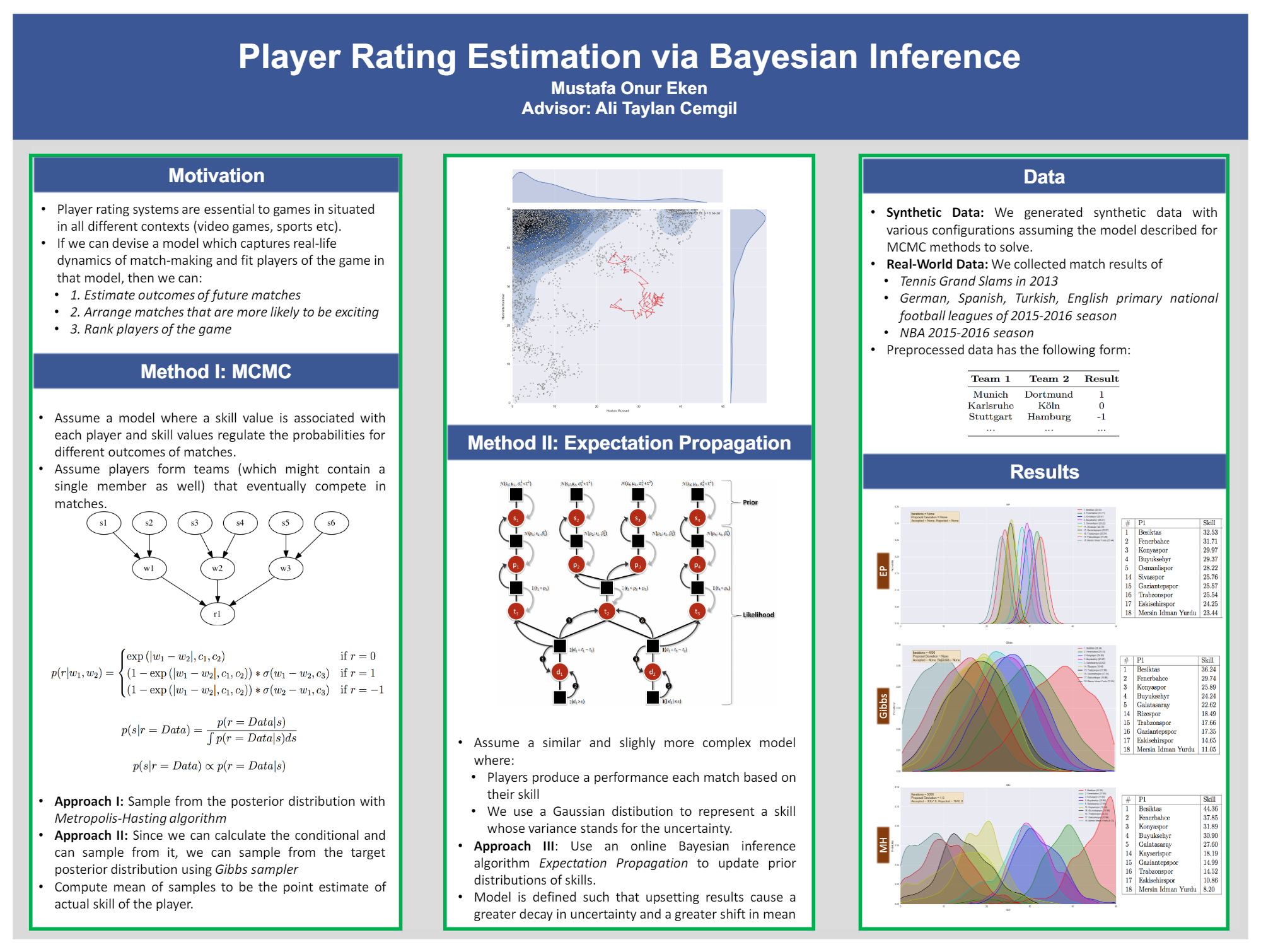
Player rating systems are essential nearly to any game situated in different contexts (video games, sports etc). This need arises from the fact that an unbalanced match is not enjoyable to any of it’s competitors. In this project we assume a generative model responsible for creation of past match results and we attempt to infer approximate posterior skills distributions by using three different algorithms: Metropolis-Hastings, Gibbs Sampler and Expectation Propagation.